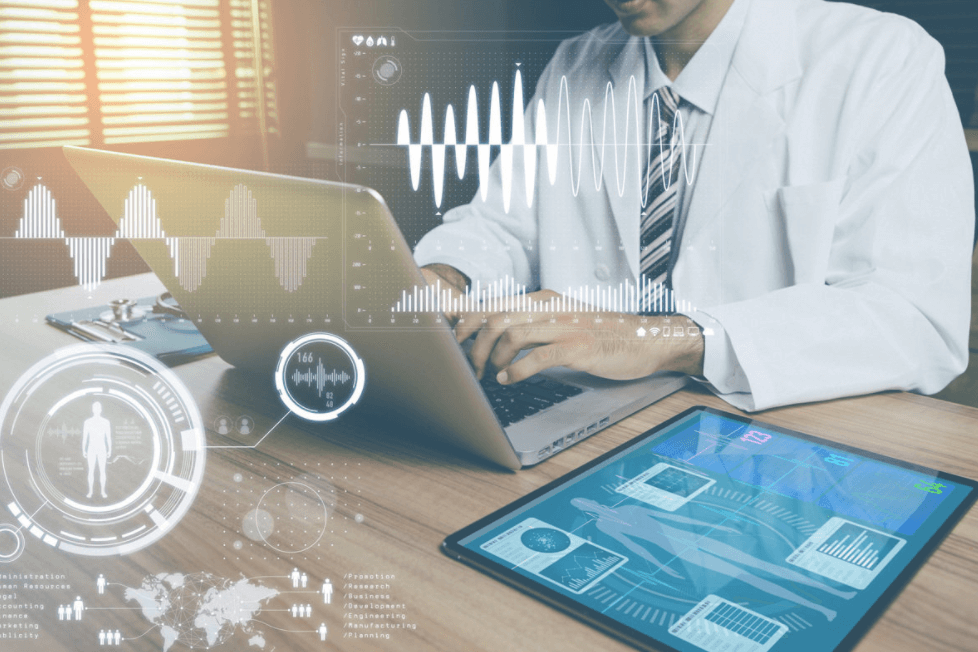
In this technological environment in which we live one can easily get lost both personally and in the corporate field and try to find the best way to practice the up-to-date versions. On the way to be successful in business, we must discover how we can leverage existing new business positions and products with existing data science systems. Before you use technology, it’s important to have a good awareness that in what way knowledge could advance products as well as make them more accessible. Because of the content around all the new versions of the technology, it can easily get off the ground and jump right on the bar without actually focusing or directing your action.
Steps to Create a Dream Data Science Team
Close cooperation between data researchers and SMEs is the key to creating a data collection infrastructure for fast and accurate decisions. Understanding the role of data scientists is important for building an “ideal team” of data scientists. There are certain steps you need to take to set up and implement data science in business as well as create a dream group to ensure ultimate success.
Centralized Approach
In the centralized model, data researchers are members of a central group and report to the head of science or data analysis. Small businesses can have 2 or 3 data researchers, but large organizations can employ dozens or even hundreds of data researchers at the Center of Excellence (COE). Having a primary scientific group allows organizations flexibility in allocating funds for a variety of tasks, exposing data researchers to a variety of analytical challenges. The centralized model also allows for the documentation and classification of best practices and ensures effective reproduction of work and teamwork. The main challenge for the basic model is that it can be difficult to assess and respond to future data science applications. This is especially true for small, centralized IT teams, which are responsible for supporting many productions or sales teams that can manage specific applications and long-term projects that require a significant investment of resources.
Risk Management
There are risks in every company’s policy. To protect the company and its assets, the management board and the senior management board assume internal and external risks. By disseminating and applying ethics, internal risk can be reduced. Your company must have a risk management plan that is specific to the risks associated with this policy. External risks are more difficult to predict and manage (such as natural disasters and economic shocks). Managers and teams should be given priority to recognize these risks and mitigate their effects. At the heart of risk management and governance is the establishment of risk control committees, the promotion of a culture of awareness, risk identification and management, and teamwork in risk management.
Communication
One of the most important opportunities is a successful business relationship. The analyst must be persuasive, whether he understands business needs or problems to be addressed, seeking additional information from stakeholders, or communicating information. However, data advertising gears are extensively used in graphic guides and facilitate the involvement of all members of the organization.
Team Work
Teamwork is another hat closure, without which practitioners cannot. Although they seem to be able to work independently, they are closely connected at different levels of the organization. The philosophy of organizations can certainly not be the culture of a separate group based on data science; in its place, the group should apply the same characteristics across the organization to maximize the information gathered from different departments.
Make Informed Decisions
A data scientist does not draw conclusions, make decisions, or make choices without sufficient data. Researchers need to decide on their position in their clients ’businesses, as well as many other things.
Conclusion
Today, data researchers have become a competitive labor market. The salary level could range from six figures to an estimated 700,000 vacancies by 2022. There are also good reasons to increase demand. The task of data scientists is to process a large amount of data embedded in data, which can then be used for a variety of business purposes, from detecting fraud to acquiring knowledge of the face. Far from being homogeneous, the field of data science today is as diverse and diverse as the business goals it helps achieve.
Awareness of this is a key element in building a research team; specialized (and atypical) skills are required for success. The specialized and complex nature of working on data knowledge is a major challenge. There is still confusion in the market about the real meaning of the term “data scientist”. Summon up, there are many benefits to understanding how technology works for your business. On account of this, it is crucial to learn data science in order to deal with the competitive industry.